That very much depends on your objective function. If you know that your objective function is highly multi-modal and complex, then BFGS is only going to give you a local minimum. If that is enough for you, then all is good. Otherwise - if you need a global optimum or simply an extremely good local minimum/maximum, then the problem becomes much more intractable as you're looking at using global optimization algorithms. Depending on the size of your problem - in terms of number of variables - it can range from relatively simple to almost impossible to locate a global optimum. On the other hand, convex objective functions shouldn't pose any difficulty on BFGS (or other algorithms) in any dimension.
That said, if we consider the BFGS method against a relatively common test function (the Rosenbrock or "Banana" function), we can take a look at this code:
import numpy as np
import scipy.optimize as optimize
import matplotlib.pyplot as plt
def rosen(x):
global fun_calls
fun_calls += 1
return np.sum(100.0*(x[1:] - x[:-1]**2.0)**2.0 + (1 - x[:-1])**2.0)
def rosen_gradient(x):
xm = x[1:-1]
xm_m1 = x[:-2]
xm_p1 = x[2:]
der = np.zeros_like(x)
der[1:-1] = 200*(xm-xm_m1**2) - 400*(xm_p1 - xm**2)*xm - 2*(1-xm)
der[0] = -400*x[0]*(x[1]-x[0]**2) - 2*(1-x[0])
der[-1] = 200*(x[-1]-x[-2]**2)
return der
def main():
global fun_calls
nvars = np.arange(2, 101)
nfuns = []
for n in nvars:
x0 = -3.14159265*np.ones((n, ))
lb = -5.0*np.ones_like(x0)
ub = 10.0*np.ones_like(x0)
bounds = zip(lb, ub)
fun_calls = 0
out = optimize.fmin_l_bfgs_b(rosen, x0, fprime=rosen_gradient, maxfun=10000)
nfuns.append(fun_calls)
m, q = np.polyfit(nvars, nfuns, 1)
fig = plt.figure()
ax = fig.add_subplot(111)
ax.plot(nvars, nfuns, 'b.', ms=13, zorder=1, label='Function Calls')
ax.plot(nvars, nvars*m+q, 'r-', lw=2, zorder=2, label=r'Fit: $y = %0.2f\cdot x + %0.2f$'%(m, q))
ax.legend(loc=0, fancybox=True, shadow=True, fontsize=16)
ax.grid()
ax.set_xlabel('Problem Dimension', fontsize=16, fontweight='bold')
ax.set_ylabel('Function Calls', fontsize=16, fontweight='bold')
ax.set_title('Rosenbrock Function', fontsize=18, fontweight='bold')
plt.show()
if __name__ == '__main__':
main()
This code will produce this picture (at least for me):
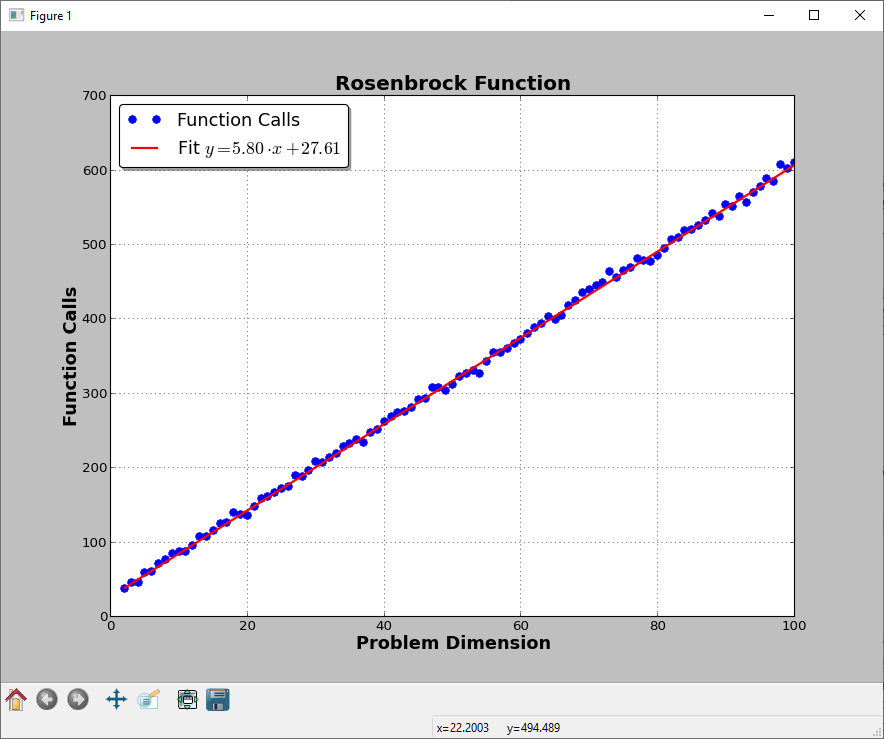
Note that I have provided also the gradient of the objective function. This picture will of course vary depending on the function itself. There are also test objective functions in the literature for which the global optimum becomes exponentially more difficult to find as the dimension increases. That said, the curse of dimensionality is almost unavoidable in nonlinear optimization.
EDIT
I have done some more tests, just for fun, with other test functions. I don't have analytical gradients for those but I used the Python package autograd (https://github.com/HIPS/autograd) to get them (and carefully counting which objective evaluations come from L-BFGS-B and which ones from autograd).
Surprise surprise: there is not that much of a pattern:
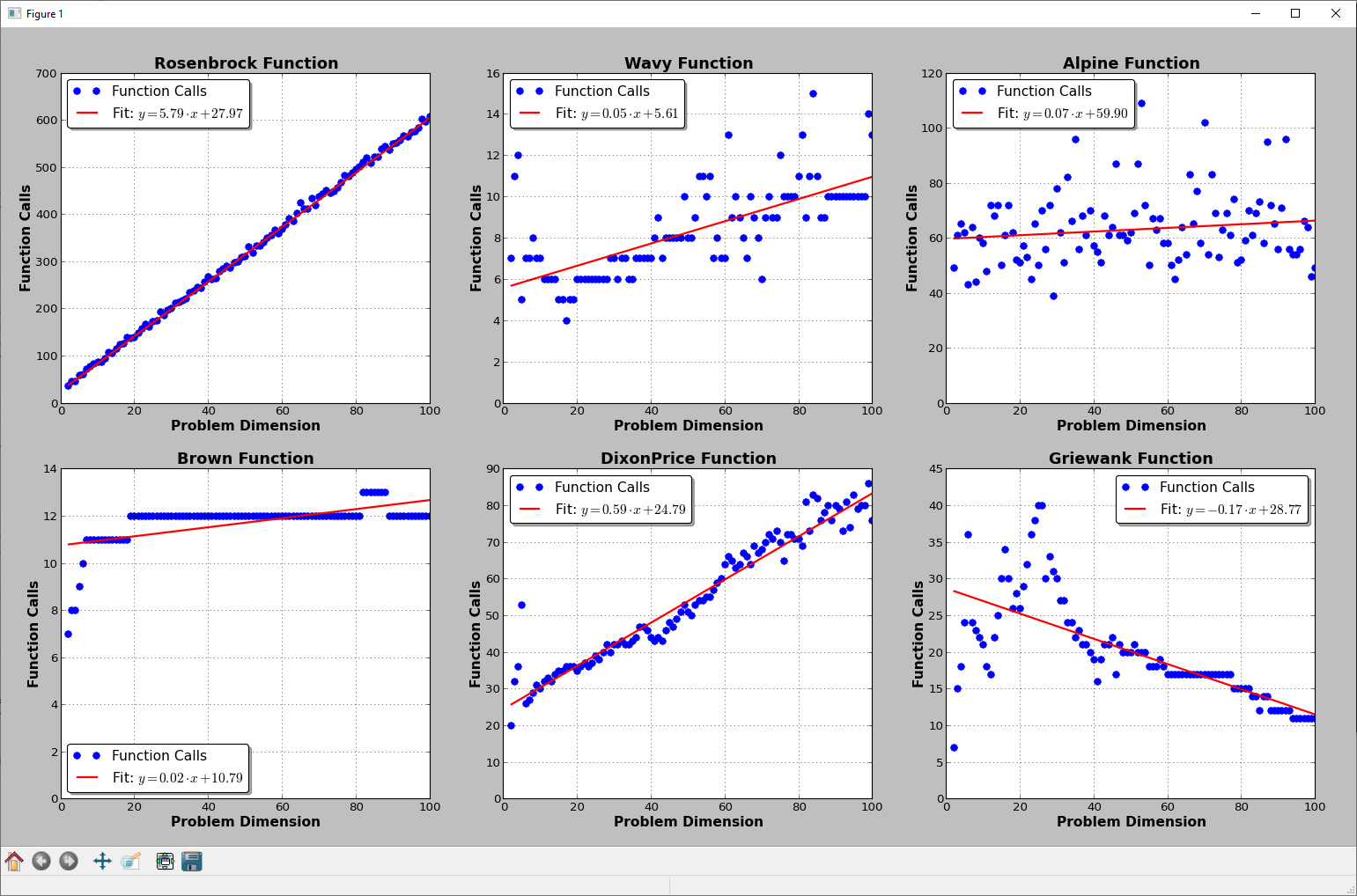
2D representation of the test functions from my work on Global Optimization:
Rosenbrock
http://infinity77.net/go_2021/scipy_test_functions_nd_R.html#go_benchmark.Rosenbrock:

Wavy
http://infinity77.net/go_2021/scipy_test_functions_nd_W.html#go_benchmark.Wavy:
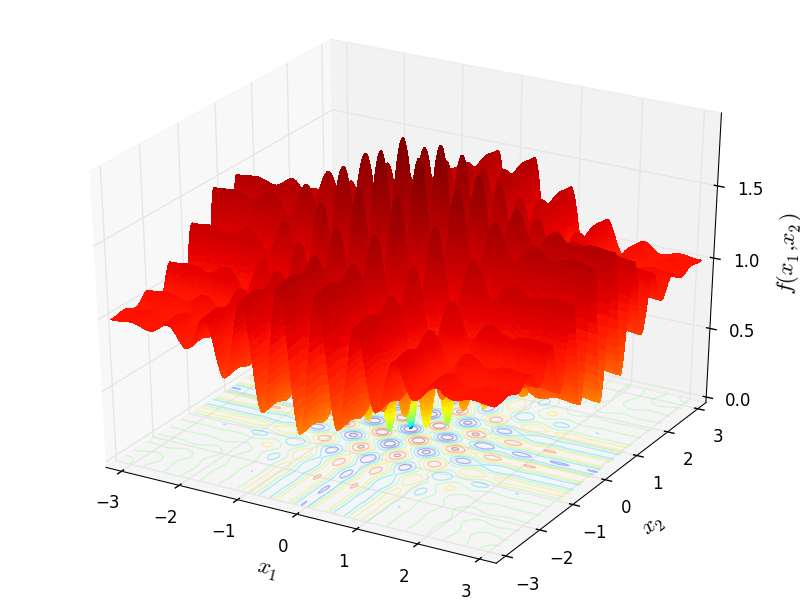
Alpine
http://infinity77.net/go_2021/scipy_test_functions_nd_A.html#go_benchmark.Alpine01
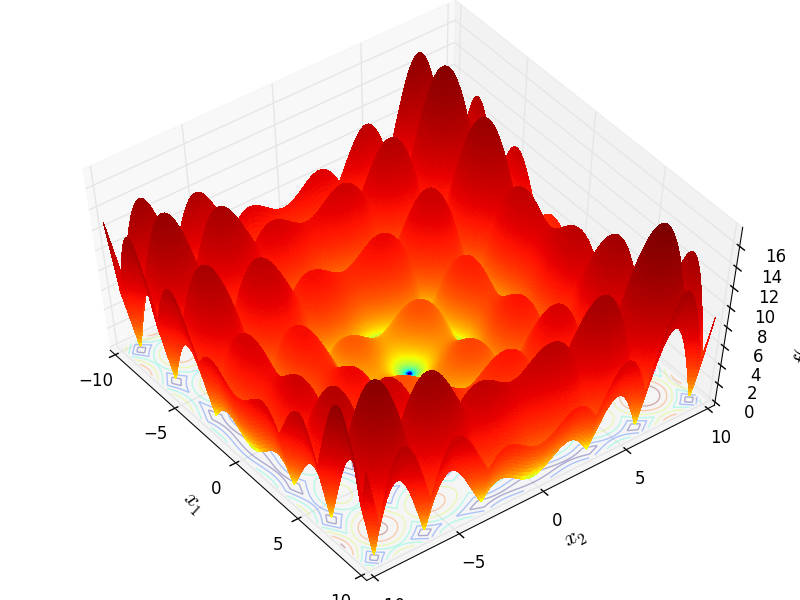
Brown
http://infinity77.net/go_2021/scipy_test_functions_nd_B.html#go_benchmark.Brown
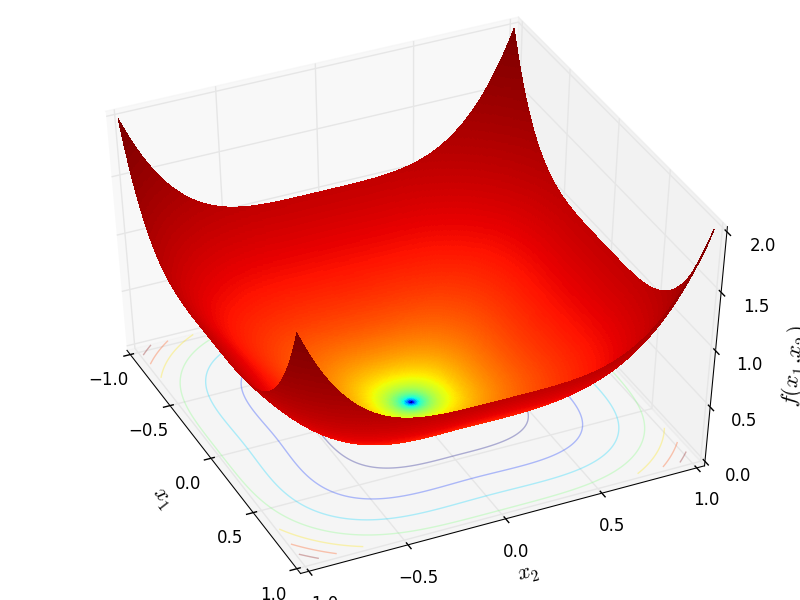
DixonPrice
http://infinity77.net/go_2021/scipy_test_functions_nd_D.html#go_benchmark.DixonPrice
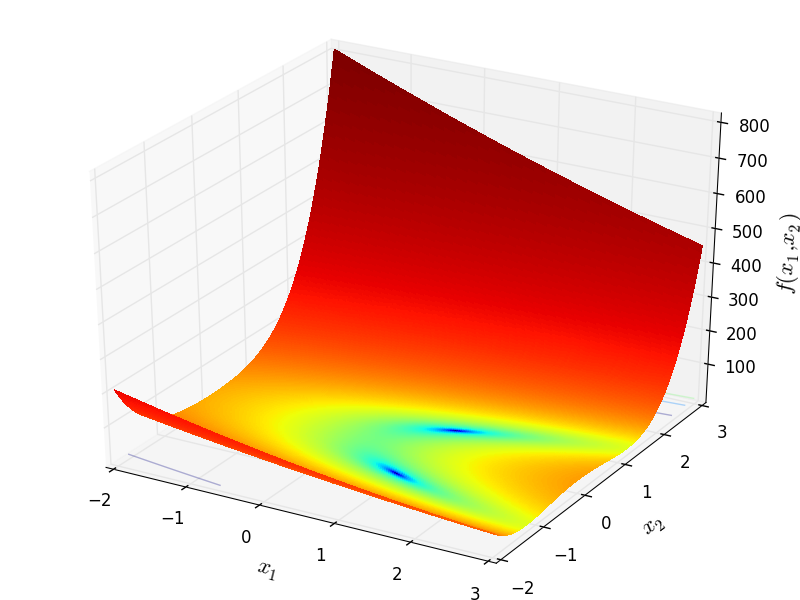
Griewank
http://infinity77.net/go_2021/scipy_test_functions_nd_G.html#go_benchmark.Griewank
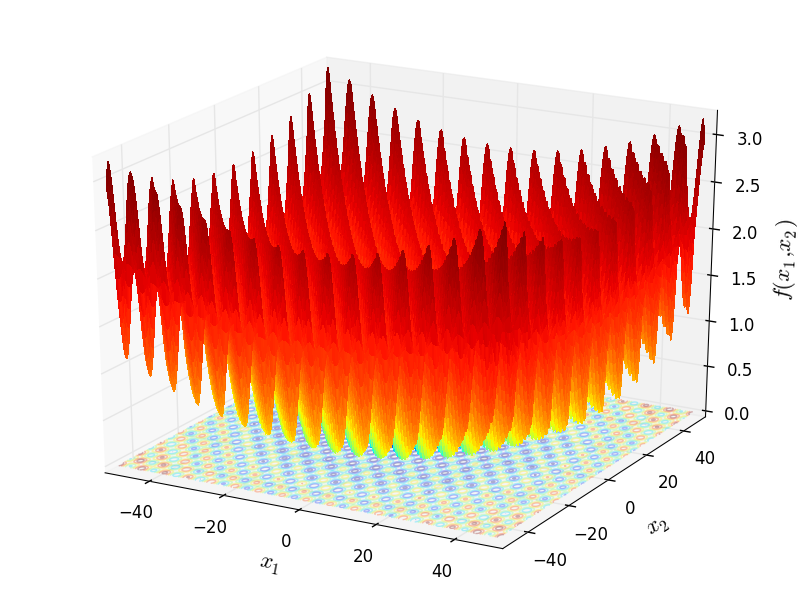
Enjoy :-)